Reinforcement Learning in Micro- and Smart Grids: Safe, Data-Driven Operating Strategies for Complex Energy Systems
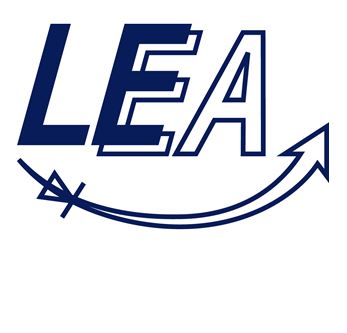
Reinforcement Learning in Micro- and Smart Grids: Safe, Data-Driven Operating Strategies for Complex Energy Systems
The research project is funded and supported by the Committee for Research and Junior Academics at Paderborn University. It was awarded the research prize of the university in 2019.
Project acronym: RLMG
Funding period: 1 year (2020/01/01 – 2020/12/31)
Project partner: Department of Intelligent Systems and Machine Learning
Project profile: https://www.upb.de/en/research/fk/research-award-winners/
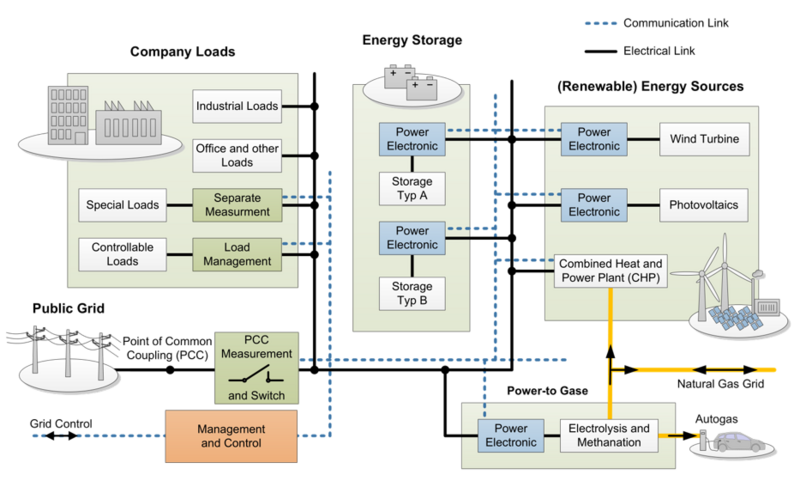
Motivation
The transformation of the current energy system to a sustainable structure characterized by renewable energies is a central social challenge of the 21st century. To achieve this, the inherent volatility of renewable energy sources requires a move away from conventional, hierarchically structured top-down energy networks towards flexible, cross-sectoral and intelligent energy systems. Therefore, in the course of the energy transition, so-called micro- and smart grids (MSG) represent an important solution component to ensure a clean, efficient and cost-effective energy supply in the future. MSG is the concept of a local grid consisting of energy sources (e.g. wind power), energy storage (e.g. battery) and energy consumers of different sectors (e.g. electricity, heat, mobility). The local integration of regenerative energies by means of MSGs, e.g. within industrial companies or residential areas, relieves the energy networks and, thus, reduces the need for cost- and resource-intensive network expansion.
The central hurdle to the establishment of MSGs is safe operation. MSGs are highly heterogeneous, complex and have a significant stochastic component, which is caused by the uncertainty of consumer behavior and renewable power plants. Classical methods of control engineering are not considered to be effective here. In contrast, reinforcement learning (RL) is a data-driven operating concept from the field of machine learning (ML), which has been able to celebrate promising success with similarly complex and stochastic problems (e.g. stock exchange trading). Nevertheless, research into MSG-specific ML strategies is still fraught with a high risk, since the security and availability of energy networks must meet the highest requirements: even a single wrong decision can lead to a complete system failure (black-out). In the absence of mathematically provable guarantees, the use of adaptive, data-driven methods of ML, whose behavior is fundamentally unpredictable, is extremely challenging in this context.
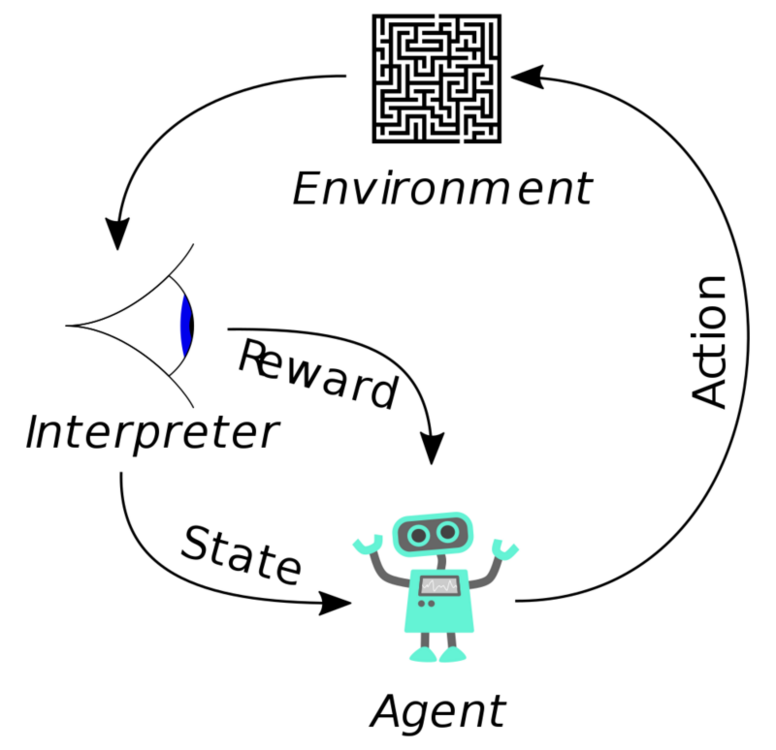
Project Objectives
The project should answer the following questions: Are RL-based operation strategies in principle able to control complex, heterogeneous and stochastic MSGs under highest security and availability requirements? Which MSG-specific methodological extensions are to be developed for reinforcement learning in order to achieve this goal?
There are basically two possible variants for operating MSGs. In the centralized approach, an agent (controller) derives control commands for all components in the MSG (e.g. battery storage) based on information about the entire system. In contrast, in the distributed concept, each entity decides on its own operating behavior (multi-agent system, MAS). This approach tends to be more robust and reduces the risk of a total failure as it is not dependent on a central component. However, the large number of decision makers increases system complexity, which increases the risk of wrong decisions in MAS as a whole.
In the course of the project, both approaches are to be examined with regard to their suitability, since no statements can yet be made on the basis of the available literature on related tasks. In order to achieve the primary goal of safe MSG operation, existing RL methods have to be extended in such a way that the learned models meet certain boundary conditions and fulfil given guarantees. In the case of decentralized control, this means the development of safe methods of MAS-RL, a very difficult problem for which there are no established approaches in the literature so far. In addition, further objectives are to be included in the investigation, e.g. minimizing operating and investment costs or maximizing regenerative energy to minimize environmental pollution. This goes hand in hand with the extension of RL methods to the case of multi-criteria optimization, for which no viable solution concepts exist so far either.
For numerical investigations a scalable model environment representing different MSG topologies is created. The goal is to automatically design arbitrary MSGs in order to evaluate the RL strategies under investigation with respect to their practicability and generalizability. In the long term, a transfer to the real test environment Microgrid-Laboratory, which is currently under construction in the LEA department, is planned.