Self-Optimizing and Adaptive Model Predictive Control of Electrical Drive Systems
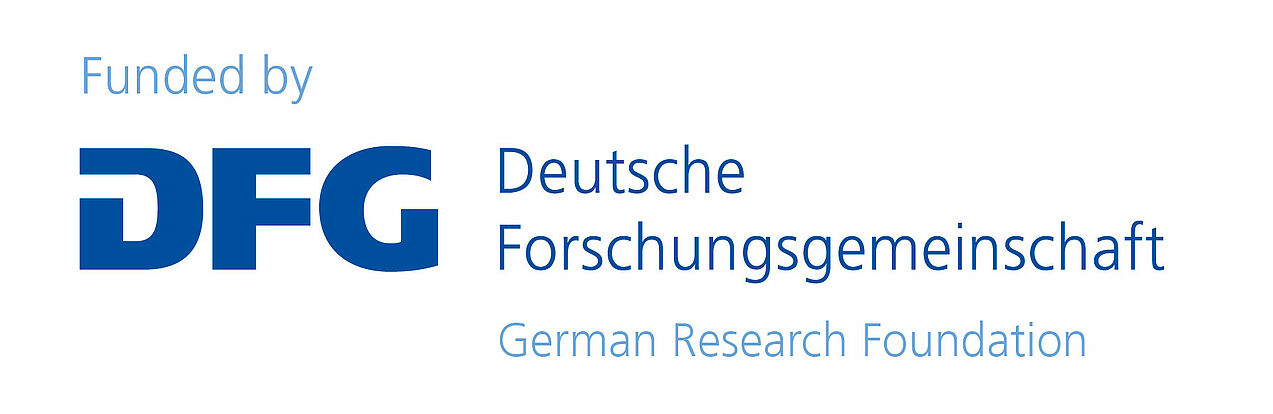
Project acronym: DFG-SelfOpt-MPC
Project period: 3 years (2018/10/01 – 2021/09/30).
Project partner: German Research Foundation (DFG) under the reference number BO 2535/20-1
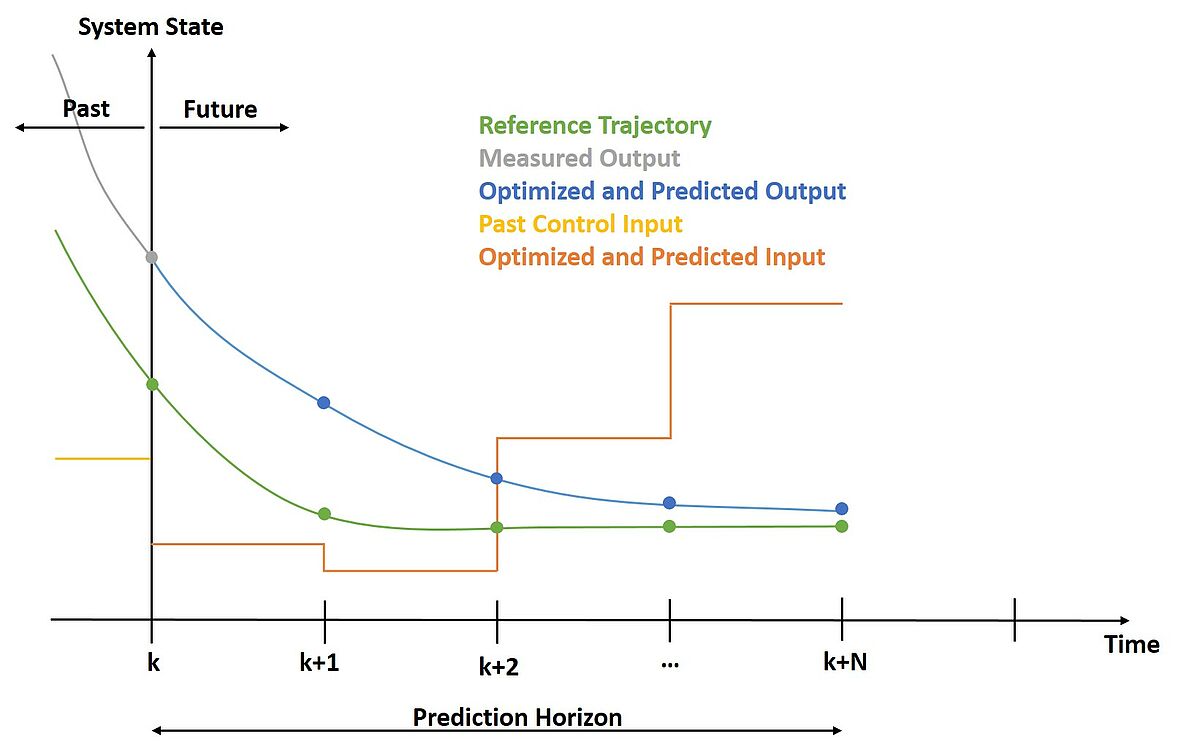
Motivation
To fulfil process-technical tasks, electric drives are used in automated production lines, power plants, centrifuges and cranes as well as in road and rail vehicles. Usually, the drives are operated in closed-loop control, whereby in addition to the actual fulfilment of the control objective, secondary aspects such as loss-reduced or sensorless operation are increasingly desired or even demanded by the operators. The state of the art is represented by innovative approaches in singular fields of control methods for electrical drives. Among others, advanced methods for current and torque control [1], temperature estimation [2], parameter identification, optimized pulse patterns [3] or self-commissioning of electrical drives can be mentioned. Typically, these methods are only applied to individual problems for specific applications. In some cases, it was possible to combine several methods for one specific application, e.g. artificial neural networks with particle swarm optimization for temperature monitoring, in order to further increase the quality, efficiency and reliability of the results obtained so far [4]. A holistic control concept, which combines state-of-the-art methods as solutions for specific subproblems, has not yet been developed.
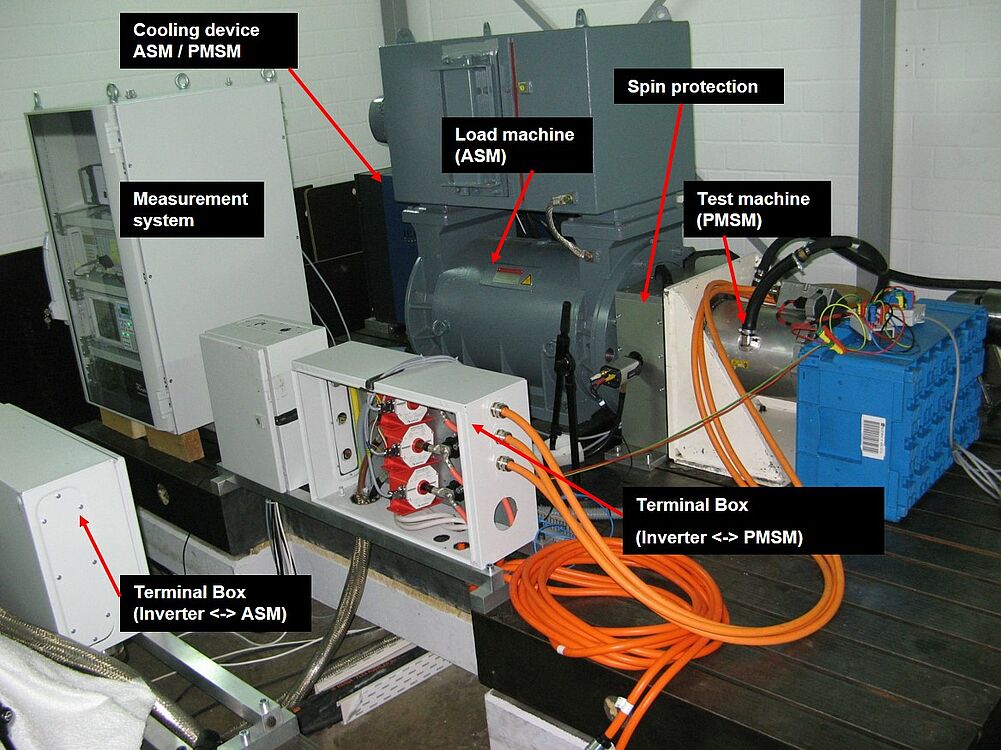
Project goals
A comprehensive generalized control concept for permanent magnet synchronous motors based on adaptive model predictive control with the following features shall be developed
- Modelling of the control loop comprising controller, motor, inverter and load
- Design and implementation of a current controller with online optimized adaptive pulse patterns for reduced motor und switching losses
- Design and implementation of a superimposed self-commissioning torque controller
- Development and integration of an online parameter identification method to adapt further control loops to improve controller performance
- Development and integration of a temperature estimation algorithm for model predictive derating to prevent thermal overload or destruction of the electrical drive
The aim is to achieve high energetic efficiency of the electric drive through the features described above. In addition, the adaptive model-predictive approach is intended to maximize control flexibility. For this reason, the controller should be able to adapt itself optimally regardless of the application, e.g. traction drives in a road vehicle or spindle drives of a machine tool. All developed algorithms will be investigated by comprehensive simulative studies. This involves a sophisticated motor, inverter and load model. In addition to that the methods will be validated by experimental studies on a test bench typical for automotive electrical drive applications (see Fig. 02).
References
[1] | M. Leuer, J. Böcker |
[2] | O. Wallscheid, J. Böcker |
[3] | K. Peter, F. Mink, J. Böcker |
[4] | O. Wallscheid, W. Kirchgässner, J. Böcker |
Contact:
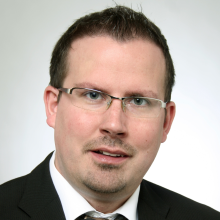
> Leistungselektronik und Elektrische Antriebstechnik (LEA)
Leiter - Vertretungsprofessor - Vertretungsprofessor Leistungselektronik und Elektrische Antriebstechnik
Büro: E4.310
Telefon: +49 5251 60-3881
E-Mail: schafmeister@lea.uni-paderborn.de
Web: Homepage