Model Predictive Direct Torque Control of Permanent Magnet Synchronous Motors
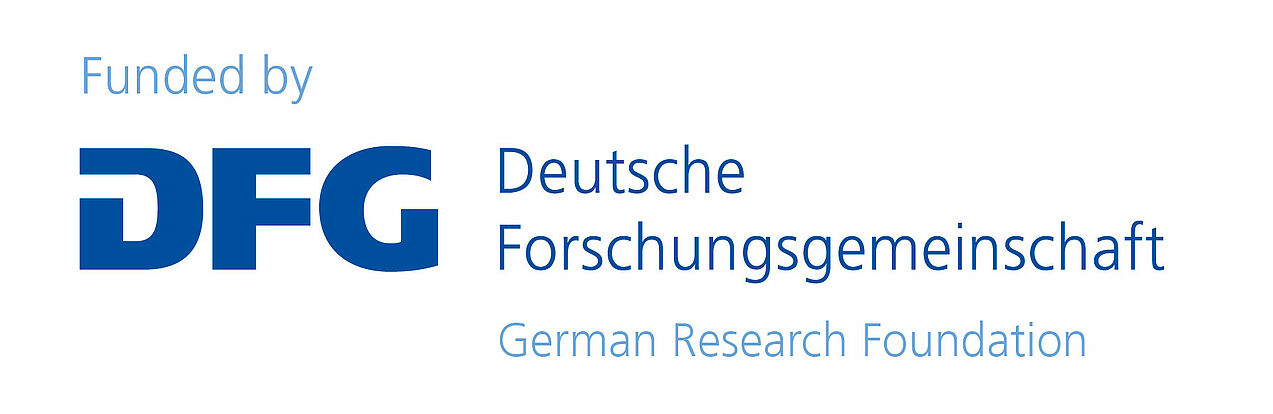
Project acronym: DFG-MPDTC
Project period: 3 years with extension (2016-2020).
Project partner: German Research Foundation (DFG) https://gepris.dfg.de/gepris/projekt/316493223
Motivation
Currently, permanent magnet synchronous motors with buried magnets (IPMSM) are mainly used for electric and hybrid vehicles due to their high power and torque densities as well as their high efficiency. In order to utilize the full performance potential of an electric drive, an adequate drive control is essential. Conventional drive controls for IPMSM are currently based on PI controllers. In comparison, Model Predictive Controllers (MPC) have the potential of a significantly better performance: A MPC exploits a process model of the system to be controlled. Based on this model optimal future control variables are determined in every sampling step. However, this online optimization sets high demands on the computation hardware. Due to innovative approaches and nowadays available more powerful computing hardware, MPC can already be used for IPMSM, but these approaches lead to other problems like high current and torque ripples.
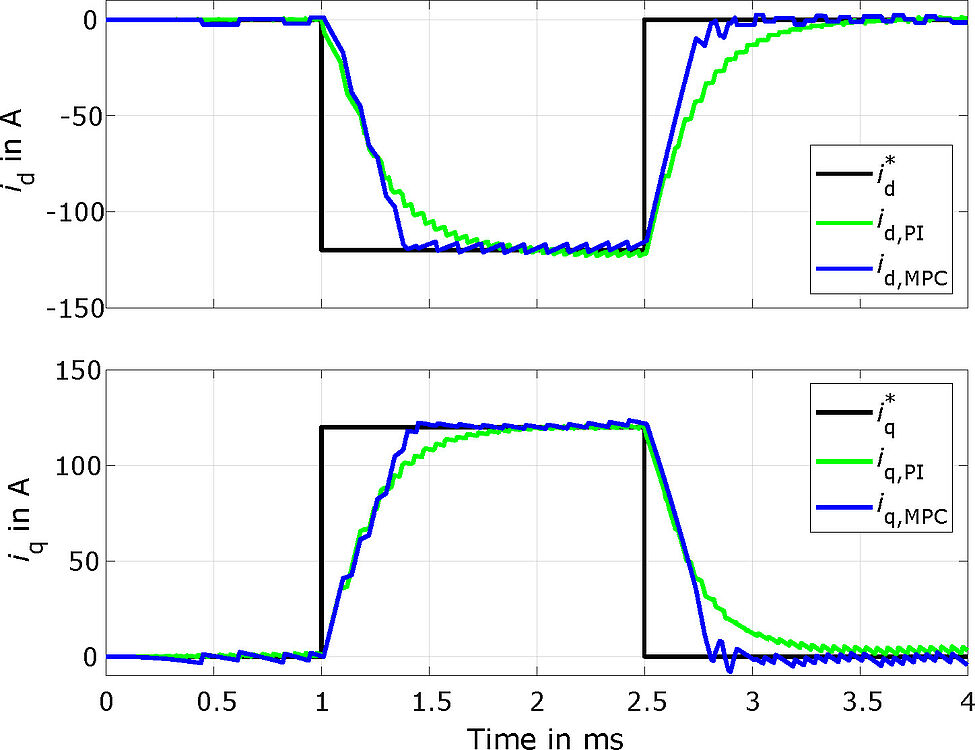
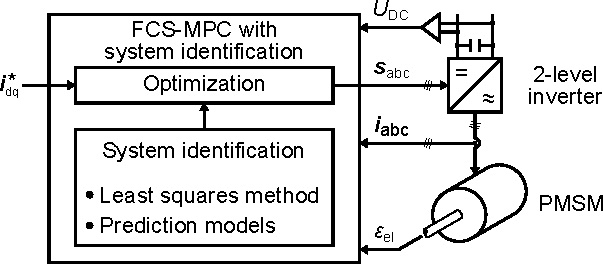
Project goals
The aim of this project is to develop an MPC approach which is specifically designed for the control of IPMSM in the drivetrain of automobiles. Therefore, the MPC approach to be developed is based on Direct MPC (also known as finite-control-net MPC). In contrast to classical MPC, only the feasible voltage vectors of the inverter (according to possible switching states) are taken into account during the online optimization. Thus, the optimization is performed very resource-efficient, so real-time MPC can be implemented on inexpensive computation hardware. Since the calculations can be easily parallelized, FPGAs or a multi-core architecture are qualified for the implementation.
The MPC will be implemented as a Direct Torque Model Predictive Control whereby the operating point selection is directly included in the inherent optimization algorithm of the MPC. This operating point optimization contributes inter alia to minimize losses. To reduce the ripples of the current and torque, respectively, the pulse pattern generation is redesigned: It can be optimized similarly to vector modulation, depending on the objective function values. This leads to a reduction of the current ripple in spite of a relatively large controller cycle time so that the computing effort can be significantly reduced thereby. By using a self-optimization for weighting the objective function, the torque dynamics for each operating point is optimized during runtime.
Nevertheless, to exploit the full performance potential of MPC-based control, an accurate process model of the system is required that takes into account (cross-)saturation or temperature dependencies as well as effects like flux harmonics or inverter nonlinearities. Therefore, a special research focus lies on the use of online identification methods to adapt the models to the behavior of the system in a data-driven approach. This also includes the comparison of different modelling approaches.
References
Contact:
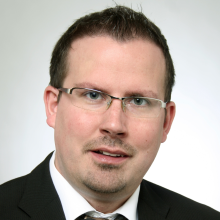
> Leistungselektronik und Elektrische Antriebstechnik (LEA)
Leiter - Vertretungsprofessor - Vertretungsprofessor Leistungselektronik und Elektrische Antriebstechnik
Büro: E4.310
Telefon: +49 5251 60-3881
E-Mail: schafmeister@lea.uni-paderborn.de
Web: Homepage