Energy Management in Vehicle Applications
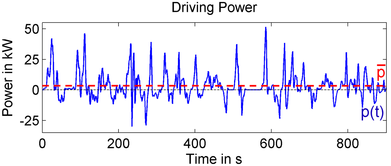
The power specifications of electric cars and traction applications are characterized by short periods of high peak but only a low mean power when accelerating and braking. So the requirements on the energy storage are on the one hand a high power density to cover the peak power, on the other hand a high energy density to cover the basic energy demands for long operation.Further requirements are a high life expectancy, low maintenance costs and –as the storage is installed on a vehicle- a low weight and volume.
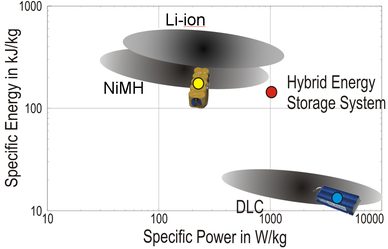
Available Electrical Energy Storages
Available electrical energy storages for vehicular applications are accumulator batteries and double layer capacitors (DLC). Batteries offer a high energy density but only a low power density and limited number of cycles, thus they are ideal long-term storage. On the contrary, DLCs offer a high power density and nearly unlimited number of cycles, so they are an ideal short term storage. The problem is that both storage technologies offer either a high power or a high energy density, but each meet only one demand at a time. So the combination to a hybrid energy storage system is advantageous. From the parallel structure of the HES, a degree of freedom arises for the distribution of the power to both storages so that an operating strategy or energy management is necessary.
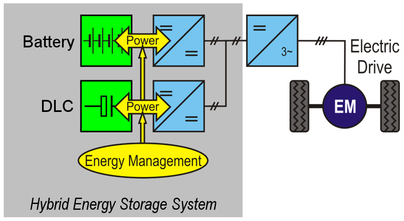
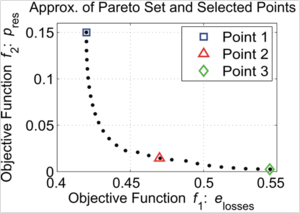
Efficient Storage for Electrical Energy: Hybrid Energy Storage System
We combine two complementary storage technologies:
- Batteries: long term storage, high energy density
- DLC: short term storage, high power density and cyclability
The distribution of the drive power to both storages is determined by intelligent self-optimization operating strategies, which take variable relevance of different objectives into account.Possible objectives are to minimize the losses of the energy storage or the efficiency respectively, or the maximization of power reserve to compensate for unexpected power demands, or the life span of the storages.
Applied optimization methods are
- Continuous multi-objective optimization via GAIO (developed by Dept. of Mathematics, University Paderborn)
- Deterministic and stochastic dynamic programming
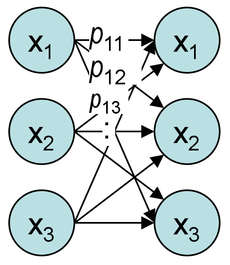
Causal Operating Strategy Considering Stochastic
One application is an electric car.
We have stochastic influences on the drive power by traffic and driver. This is displayed in the plot. This plot shows the velocity profiles of two trips on the same route. There are strong similarities, but also some stops at traffic lights which are missing in the other profile. So a suboptimal but causal operating strategy is necessary. We apply SDP, a method which models the driving cycle and power as a stochastic process. The system is described by its state, comprising velocity, power and state of charge of the short term storage, every 5 seconds. Based on recorded driving cycles, the transition probability for each state is then calculated as a homogenous Markov chain. This means that future states only depend on the actual, not on former ones and thus are time and position independent. The cost function to be minimized is the expectancy value of the losses and the power reserve to compensate for unexpected power demands. The result of optimization is a look-up table which can be used online.
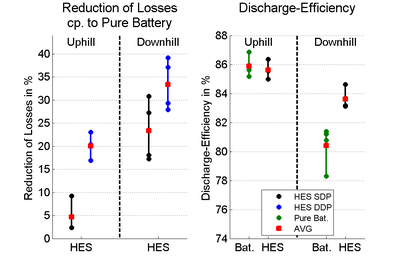
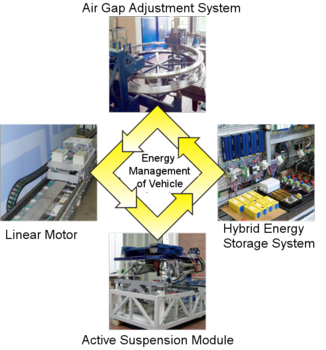
Test Setup
The causal operating strategies for an energy management have been evaluated at the following test setup:A lookup-table was calculated by SDP based on 6 power profiles recorded in urban and suburban traffic. The examined profile was not included in the database. The profiles are on the same route, but in two different directions. There is a difference in altitude of 57m. The energy storage is sized for a range of 60 km and a maximum power of 150 kW. The results show that the weight of the energy storage can be reduced by 36% by hybridization compared to pure battery storage capable of the driving power. This leads to a reduction of drive power by 6%. The losses of the storage system can be reduced by 15% on average at the same time, the efficiency can be increased by 1.4 percentage points. On the downhill cycles, a higher reduction of the losses by 23% is possible due to the higher efficiency of the HES during recuperation of 3-3.5%. Comparison to optimal results acquired by non-causal Deterministic Dynamic Programming (blue coloured in plot) shows that there is still some potential for loss reduction especially on uphill cycles. This is topic of ongoing research.
Ongoing Research
As part of the Collaborative Research Centre 614 „Self-optimizing concepts and structures in mechanical engineering” (SFB 614) the current research on energy management is focused on the self-optimizing energy management in decentralized interconnected systems. Subject of this research is the power supply system on board of the RailCab, a system of autonomous railway vehicles. As of now the energy is managed by a central unit. The current approach is to move the management to the parts of the system, each optimizing itself and the whole system according to the current situation. For this the parts of the RailCab which exist on several autonomous test beds are being interconnected to be able to negotiate an energetically optimal system state.
References:
[1] | Romaus, C.; Böcker, J.; Witting, K.; Seifried, A. & Znamenshchykov, O. Optimal Energy Management for a Hybrid Energy Storage System Combining Batteries and Double Layer Capacitors IEEE Energy Conversion Congress and Expo (ECCE), San Jose, USA, 2009 |
[2] | C. Romaus, K. Gathmann, J. Böcker Optimal Energy Management for a Hybrid Energy Storage System for Electric Vehicles Based on Stochastic Dynamic Programming Vehicle Power and Propulsion Conference (VPPC 2010), Lille, Frankreich, 2010 |
[3] | C. Romaus, K. Gathmann, J. Böcker Optimal Energy Management for a Hybrid Energy Storage System for Electric Vehicles Based on Stochastic Dynamic Programming Vehicle Power and Propulsion Conference (VPPC 2010), Lille, Frankreich, 2010 |
[4] | C. Romaus, K. Gathmann, J. Böcker Optimal Energy Management for a Hybrid Energy Storage System for Electric Vehicles Based on Stochastic Dynamic Programming Vehicle Power and Propulsion Conference (VPPC 2010), Lille, Frankreich, 2010 |
[5] | Klöpper, B.; Romaus, C.; Schmidt, A.; Vöcking, H. & Donoth, J. Defining Plan Metrics for Multi-Agent Planning Within Mechatronic Systems International Design Engineering Technical Conferences & Computers and Information in Engineering Conference, (ASME), Brooklyn, New York, USA, 2008 |
[6] | Klöpper, B.; Romaus, C.; Schmidt, A. & Vöcking, H. Gausemeier, J.; Rammig, F. & Schäfer, W. (Eds.) A Multi-Agent Planning Problem for the Coordination of Functions Modules 7th International Heinz Nixdorf Symposium -- Self-optimizing Mechatronic Systems: Design the Future, Heinz Nixdorf Institut, Universität Paderborn, 2008, 223, S. 377 – 393 |
[7] | Klöpper, B., Sondermann-Wölke, C., Romaus, C., Vöcking, H. Probabilistic Planning Integrated in a Multi-level ependability Concept for Mechatronic Systems IEEE Symposium on Computational Intelligence in Control and Automation (CICA), Nashville, Tennessee, USA, 2009. |
[8] | Knoke, T.; Romaus, C.; Böcker, J.; DellAere, A. & Witting, K. Energy Management for an Onboard Storage System Based on Multi-Objective Optimization 32nd Annual Conference of the IEEE Industrial Electronics Society (IECON), Paris, France, 2006, S.4677 - 4682 |
[9] | Böcker, J.; Huber, T.; Romaus, C. & Specht, A. Elektromobilität in Paderborn -- Kerntechnologien für Automobile von morgen ForschungsForum Paderborn, 2011, S. 14 |
[10] | Böcker, J.; Huber, T.; Romaus, C.; Specht, A. & Wallscheid, O. Kluge Köpfe unter Strom: Elektro- und Informationstechnik an der Universität Paderborn Ingenieurwissenschaften Deutschland - Zukunft & Faszination der Elektro- und Informationstechnik, Institut für Wissenschaftliche Veröffentlichungen, VDE/ZVEI, 2011, S. 274-281 |
[11] | Adelt, P.; Donoth, J.; Gausemeier, J.; Geisler, J.; Henkler, S.; Kahl, S.; Klöpper, B.; Krupp, A.; Münch, E.; Oberthür, S.; Paiz, C.; Podlogar, H.; Porrmann, M.; Radkowski, R.; Romaus, C.; Schmidt, A.; Schulz, B.; Vöcking, H.; Witkowski, U.; Witting, K. & Znamenshchykov, O. Selbstoptimierende Systeme des Maschinenbaus -- Definitionen, Anwendungen, Konzepte. HNI-Verlagsschriftenreihe, 2008, Band 234, ISBN 978-3-939350-53-8 |
[12] | DellAere, A.; Hirsch, M.; Klöpper, B.; Koester, M.; Krupp, A.; Krüger, M.; Müller, T.; Oberthür, S.; Pook, S.; Priesterjahn, C.; Romaus, C.; Schmidt, A.; Sondermann-Wölke, C.; Tichy, M.; Vöcking, H. & Zimmer, D. Verlässlichkeit selbstoptimierender Systeme - Potenziale nutzen und Risiken vermeiden HNI-Verlagsschriftenreihe, 2008, Band 235, ISBN 978-3-939350-72-9 |
[13] | Frank, U.; Giese, H.; Müller, T.; Oberthür, S.; Romaus, C.; Tichy, M. & Vöcking, H. Gausemeier, J.; Rammig, F.; Schäfer, W.; Trächtler, A. & Wallaschek, J. (Eds.) Potenziale und Risiken der Selbstoptimierung für die Verlässlichkeit mechatronischer Systeme 5.~Paderborner Workshop Entwurf Mechatronischer Systeme, 2007 |
[14] | Boxnick, S.; Klöpfer, S.; Romaus, C. & Klöpper, B. Multiobjective Search for the Management of a Hybrid Energy Storage System 8th International Conference on Industrial Informatics (INDIN), Osaka, Japan, 2010 |
[15] | Dumitrescu, R.; Gausemeier, J. & Romaus, C. Towards the Design of Cognitive Functions in Self-Optimizing Systems exemplified by a Hybrid Energy Storage System 10th International Workshop on Research and Education in Mechatronics (REM), Glasgow, UK, 2009 |
[16] | Peters, A.; Romaus, C.; Henke C. & Böcker, J. Linearantriebstechnik mit inhärenter kontaktloser Energieübertragung 6. Fachveranstaltung Kontaktlose Energieübertragung - Stand der Technik, Haus der Technik, Stuttgart, Deutschland, 2011 |